LoLSolved - League of Legends Builds and Guides using Genetic Algorithms
LoLSolved is an software based theorycrafter for League of Legends builds. Select the champion, select the role, select the high level stats you want to optimize for e.g. Burst Damage, Physical Damage Resistance, Gap Close, etc. and LoLSolved will return the optimal build order.
The builds on the site are all dynamic, you can change the Runes, Items, increase the enemy's Armor, add Grievous Wounds, start with a Seeker's Armguard, change a build to ARAM mode, etc. and LoLSolved will re-optimize the rest of the build order for you.
You can create guides from the lol builds you find in two clicks, then add wiki text and even embed your Twitch channel.
Documentation
About
Hi!
So this site is an application of my PhD work on solving complex acquisition problems to the League of Legends Rune/Item systems. It works by combining genetic algorithms with goal modelling.
Simple explanation:
Watch this video by CGP Grey on Genetic Algorithms:
And take a look at this acquisition graph:
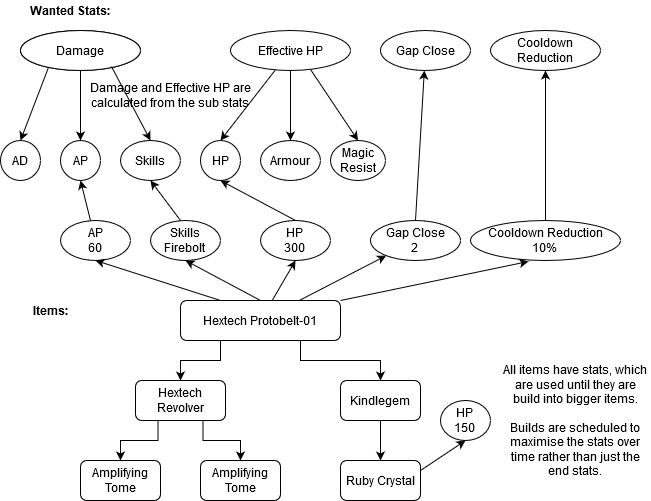
The bots wire up the acquisition graph (rather than a neural network) and teacher bot looks at the wired up acquisition graphs and rates the build by the stats they produce (over time rather than just the end stats).
We are using learning in an environment rather presenting training examples to bots. Therefore we use the factory method rather than linear algebra. You can see learning in an environment in the video below. Our bots are build orders and the environment is the League of Legend's math formulas.
Currently modelling:
- Champion Actives
- Champion Passives
- Item Actives
- Item Passives
- All basic stats
- Stats now are better than stats later
- The fully optimal build order for all items and their component items
- Effects that alter the effects of other stats
- Different collections of stats that are wanted in the different roles and positions
Example model of Hextech Protobelt-01:
{ "acquirable": 1, "costs": [ {"amount": 650, "name": "Gold"}, {"amount": -1, "name": "Slot"}, {"amount": -1, "name": "Waveclear"} ], "depends": [ {"name": "Hextech Revolver"}, {"name": "Kindlegem"} ], "name": "Hextech Protobelt-01", "provides": [ {"name": "HP Base", "providedValue": 300.0}, {"name": "Ability Power Base", "providedValue": 60.0}, {"name": "Cooldown Reduction Percentage", "providedValue": 10.0}, { "name": "Active Skills", "scriptValues": { "activeAbility": true, "apBase": [82.5, 103, 124, 151, 165], "apRatio": 0.275, "cooldown": 40000, "name": "Hextech Protobelt-01 Ability 1" } }, {"name": "Gap Close", "providedValue": 2}, {"name": "Sudden Impact Trigger", "providedValue": 1.0} ] }
Each different build contains a set of stats that are wanted with various ratios, which you can vary with the controls. Damage is measured as an average over 1.5 seconds, except for sustain builds that measure over 4 seconds and ADC builds that increase from 1.5 to 4.0 seconds as the game progresses.
Generating builds currently takes 10 minutes (per champion per build) on a 32 core AMD Ryzen Threadripper 2990WX and involves searching over 400,000 possible plans. The number of plans searched is effectively increased further by mixing populations from different champions together raising the effective number of searched builds into the tens of millions.
The thesis is on adapting genetic algorithms to allow them to be used for acquisition problems. A large general class of acquisition problems that LoL build orders happens to fall into (There is nothing LoL related in the thesis.)
Thesis downloadHelp
Introduction
This site contains computer optimized League of Legends builds.Using the Build Calculator
Build Page
To use the site go to the Builds page on the top bar.First, select your champion, you can use the search bar at the top to find a champion quickly
For now select Zoe as the champion.
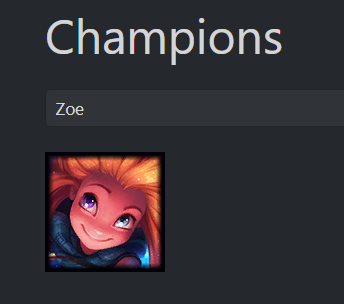
Champion Page
On the champion page, you can open the build calculator or see builds written by other people in the guides.For now open the build calculator.
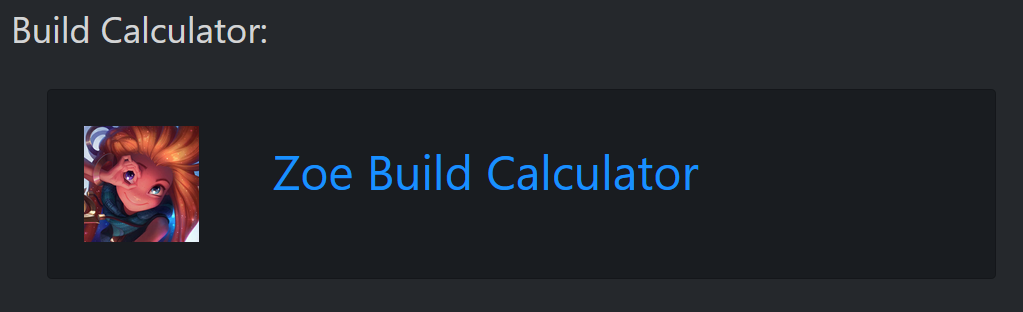
Build Calculator
You now need to select what role you are playing the champion in.
At the top is Mid, Adc, Top, Assassin, Jungle & Support.
Then you need to select the play style of the champion.
The play style determines the set of stats that the site will optimize the build for.
In this case will select Mid -> Standard Mage Builds for Zoe.

All builds are optimized for raw damage. The rest of the stats being optimized for are shown below. In this case: Ability Haste, Mana Sustain, Stasis and Gap Close. For this example we will set Ability Haste to Very High.

This gives the following build order below. That is optimized to give the stats we asked for. Stats like Ability Power, Attack Damage, Attack Speed, etc. were automatically translated into Damage using a model of Zoe's abilities.
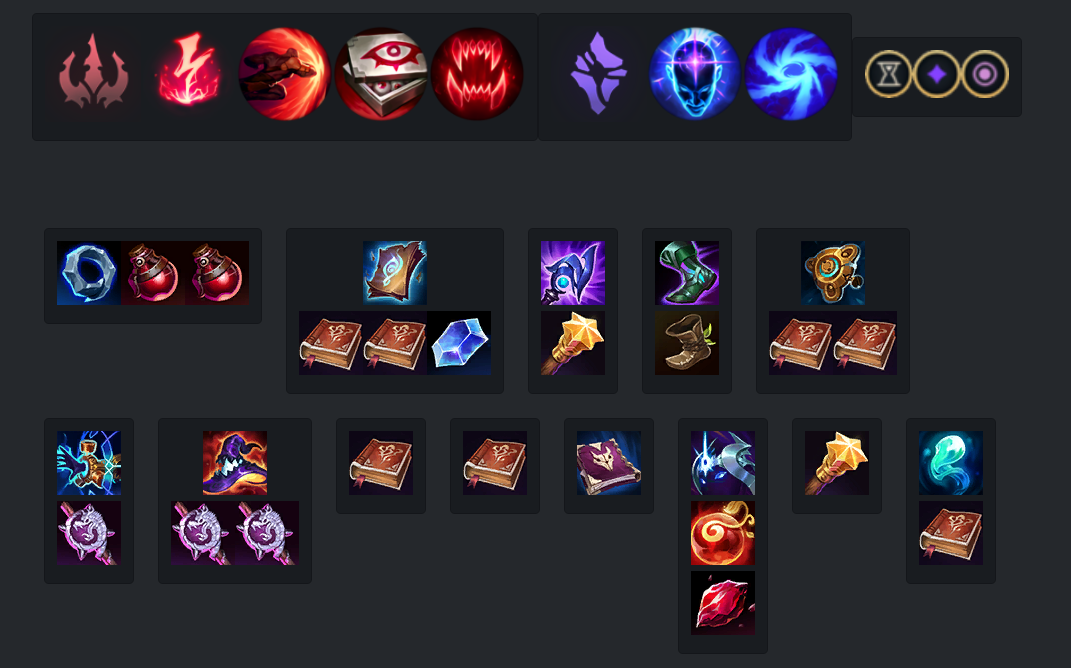
But the on paper stats don't always tell the complete picture, Zoe has a strong early game and can take advantage of this by using early game items. We can force the taking of Biscuit Delivery, Timewarp Tonic and Corrupting Potion. A bar will appear under the Reset and Add Engine Option buttons, initially Red, it will change to Yellow (once it first gets a possible build) then disappear once it has finished optimizing the build. This runs in your browser and will take 0 to 60 seconds to complete. It runs faster in Google Chrome due to it's better Javascript engine.
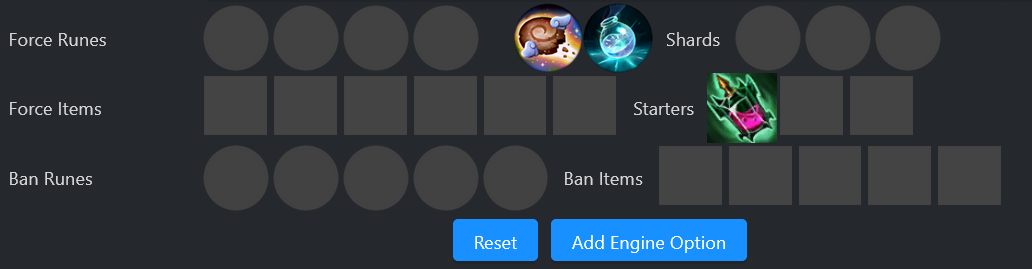
We now get the following build:
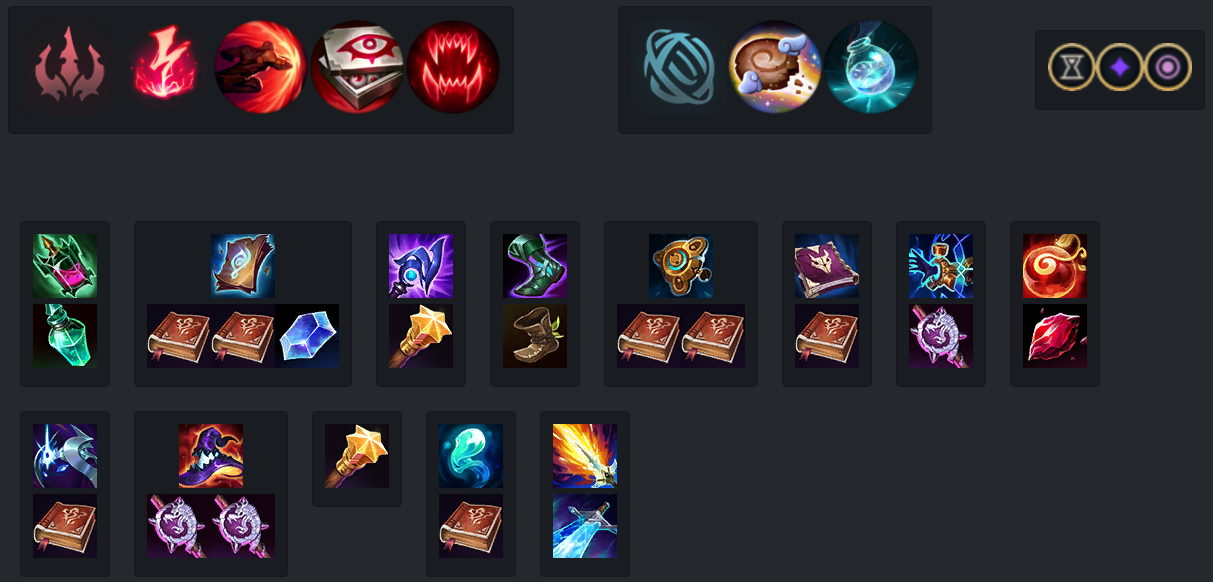
This is good but we think the boots are in the wrong place. Let's change that by using the Add Engine Option button. We can add two engine options, Set Tier 1 Boots Gold and Set Tier 2 Boots Gold to move the boots into the correct position.

This produces the following build below with the boots in the right place:
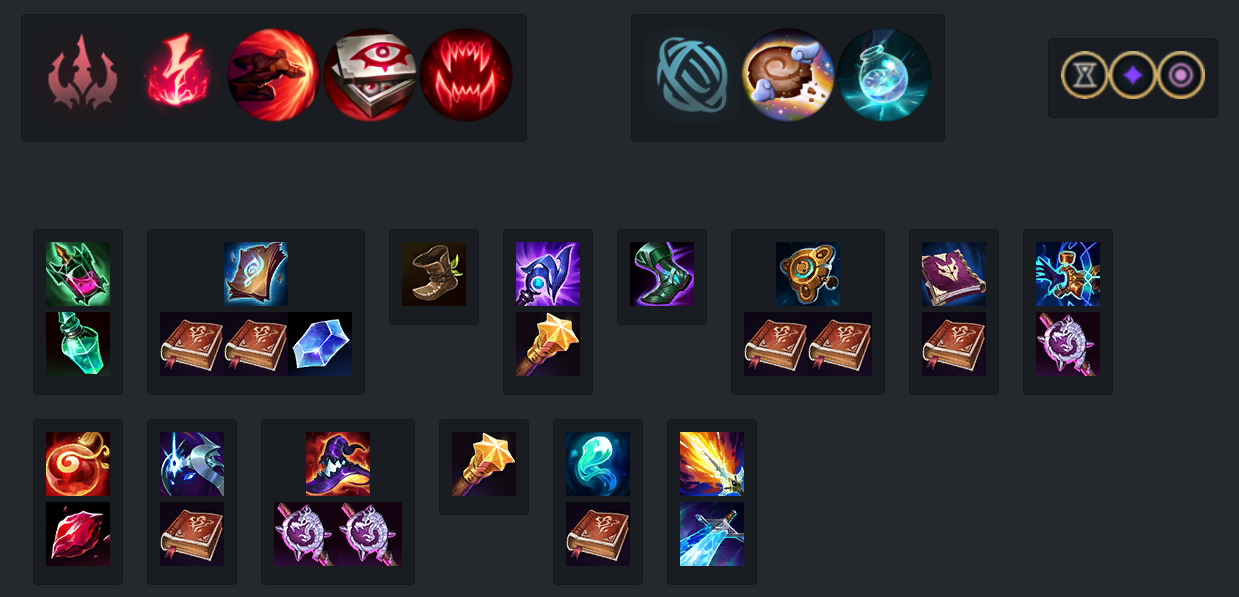
Engine Options
The optimizer supports the following options:Engine Option | Description |
---|---|
Tier 1 Boots | Sets maximum gold spend in build before getting boots |
Tier 2 Boots | Sets maximum gold spend in build before getting tier 2 boots |
Change Mana Curve | Required mana is checked at 5 points through the build order, you can adjust the required mana here. The required mana is multiplied by the values given. |
Limit Gold | Limit total build gold to X. Can be useful for working out the next item to buy |
Ultimate Build | Quads gold income after minute 20 and raises item slot count by 1 |
Add Data Files | Add additional build names here to consider items from those builds whilst searching. Important when making drastic changes to build options. Comma seperated list of data files. The default value displays the data file for the current build. Look at other builds to get the names of their data files. |
Spellblade Proc Count | Number of times spellblade will proc |
Melee Champion Auto Attack Modifier | Percentage of time a champion is typically in contact with another champion for making auto attacks. Range: 0.0 - 1.0 |
Item Groups | Consider a set of items at a position in the build Usage: Position,Item 1,Item 2,Item 3 e.g. 0,Horizon Focus,Rabadon's Deathcap |
Enemy HP | Enemy HP from start of game (minute 0) to end of game (minute 45), default: 620 to 3300 |
Enemy Armor | Enemy armor from start of game (minute 0) to end of game (minute 45), default: 30 to 110 |
Enemy Magic Resist | Enemy magic resist from start of game (minute 0) to end of game (minute 45), default: 30 to 64 |
Ban Extra Runes | If you run out of ban rune slots above, you can add an extra rune to ban here |
Ban Extra Items | If you run out of ban item slots above, you can add an extra item to ban here |
Combat Length Override | Change the combat length for damage calculations. 0.5 is Poke, 1.5 is Burst, 4.0 is Sustain |
Early Combat Length Override | Change the early combat length for damage calculations default: 0.5 for Poke Damage |
Mid Game Combat Length Override | Change the mid game combat length for damage calculations default: 1.5 for Burst Damage |
Late Game Combat Length Override | Change the late game combat length for damage calculations default: 4.0 for Sustain Damage |
Extended Combat Value | Change how effective Conqueror and DoT items are on this champion. Increasing this will encourage
the selection of Conqueror and purchase of items such as Liandry's Anguish and Demonic Embrace. Range: 0.0 - 1.5 |
Override Stat Ratios | Change the ratio between desired stats E.g. {"Burst Damage": 1, "Ability Haste": 1, "Effective HP Magic": 0.065, "Effective HP Physical": 0.082} This can be used to create builds outside of normal ranges given by the stat buttons For example, you can set Ability Haste to 10 to get a build that focuses almost completely on Ability Haste |
Evolve Icathian Rain | Evolve Kai'Sa's Icathian Rain before gold amount X is spent in the build |
Evolve Void Seeker | Evolve Kai'Sa's Void Seeker before gold amount X is spent in the build |
Evolve Supercharge | Evolve Kai'Sa's Supercharge before gold amount X is spent in the build |
Schedule Stat | Modify how much the stat counts for over time Format: [(ts1, multiplyer1), (ts2, multiplyer2), (ts3, multiplyer3)] E.g. [(0, 5.0), (1200, 0.0)] says the stat counts 5x at the start of the build, linearly scaling down to 0x at minute 20 |
Making a guide
First select the champion you want to make a guide for.
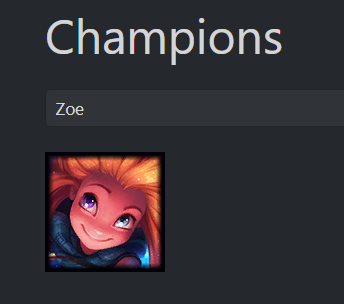
Click on Create New Guide

You will now need to make an user account to manage your guides. Just an username and password is needed. You can skip account creation by pressing the skip account creation button, which creates you a temporary guest account that can be upgraded to a full account on the account page.
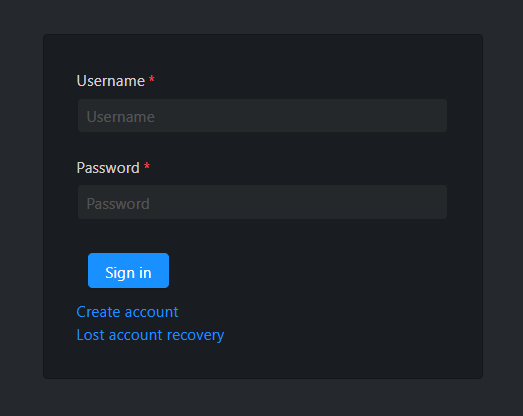
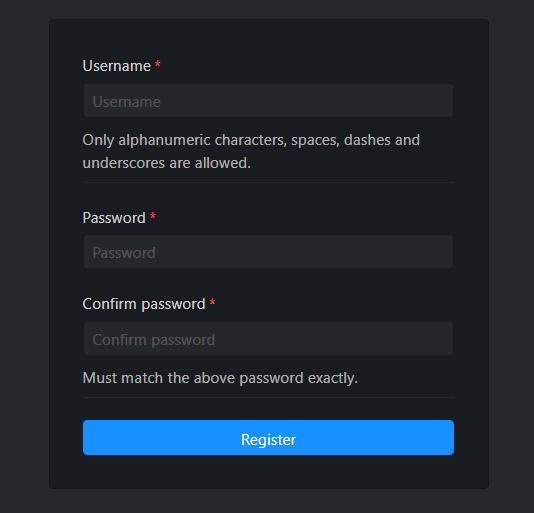
On creating an account you will be given an account recovery token in case you forget your password. If you are writing an serious guide, screenshot it and save it to your computer.
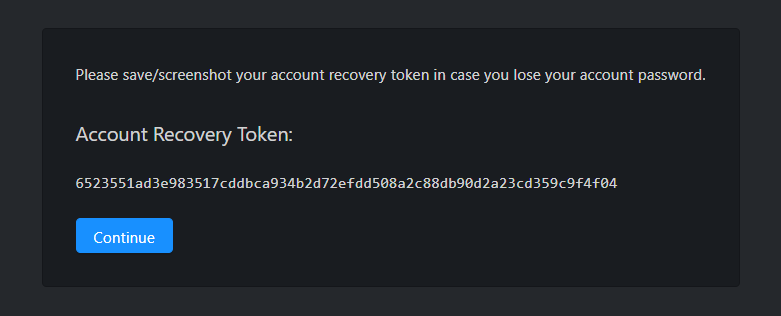
Fill out the basic info about your guide: Guide title, can other people find the guide, the guide's image in the list and the position
- Guide Title
- Is the guide public?
- Guide image when listed on the champion's page
- Guide position
If you have a Twitch Channel you can add it in "Embed Your Twitch Channel" to have it auto play on the guide. Unlike some other sites, there is no charge for this feature.
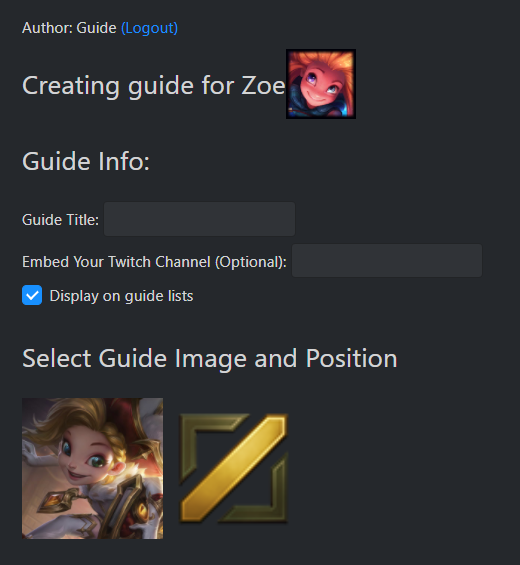
Set the summoner spells for the guide. You can have up to 4 sets.
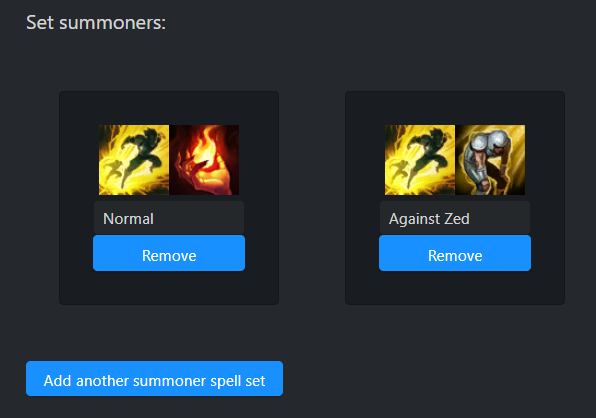
Set the skill order. Use the quick set option then manually click on the skills grid to change the skill order.
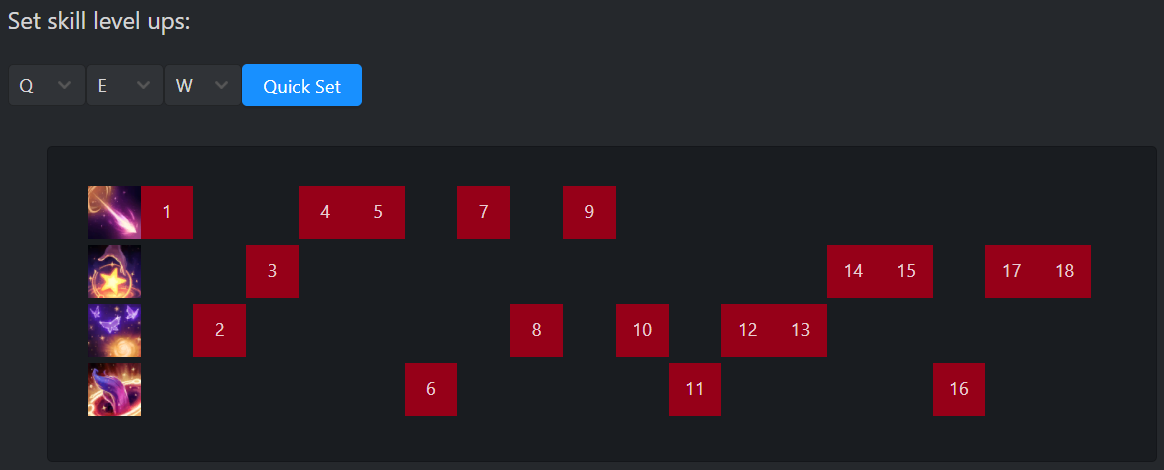
Now you can add builds from the build calculator. Click on Build ID Finder to open it in a new tab. You will need to fill in the build names and builds ID for the guide.
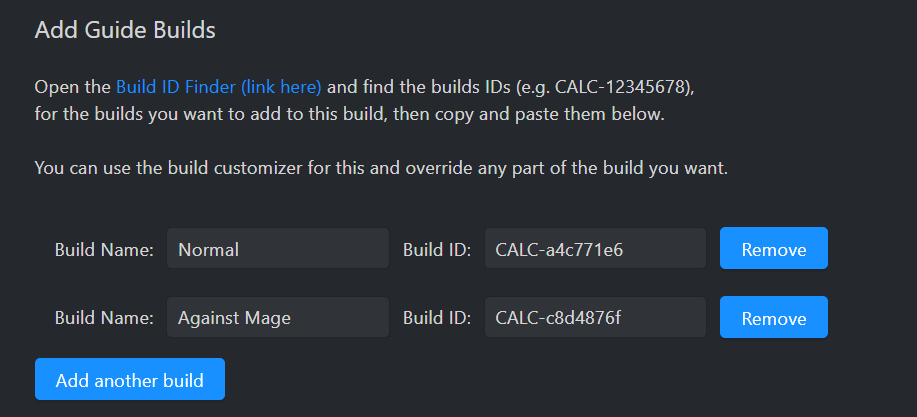
This is the build calculator but at the top is an build ID for use in guide creation.
You can make any changes you want including forcing all the runes and items to be whatever you want, which
probably defeats the point but it's up to you as the guide author.
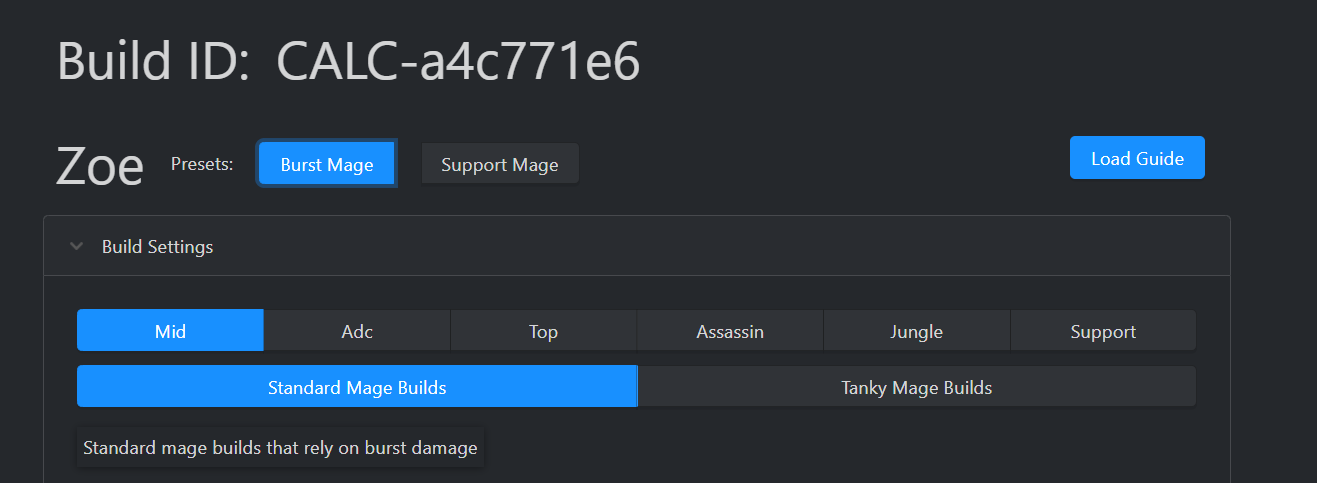
Next is the guide text. You have access to wiki style text for this. You can do most things with the UI.
More advance stuff needs you to use bbcode. (See
guide formatting instructions)
Three buttons to note are:
The LoL button that lets you insert images listed
here such as champion, skill, rune and item images.
The insert image button that lets you insert images hosted on another website.
The view source button for working with bbcode directly.
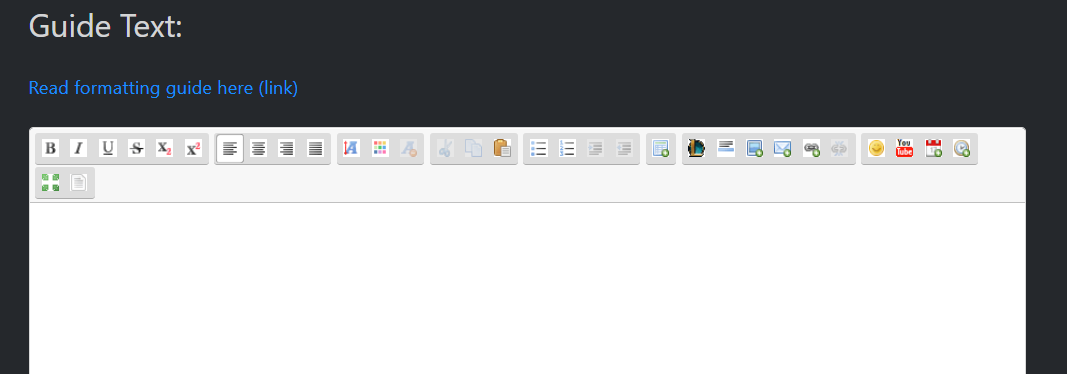
Submit your guide. Don't worry you can change it later!

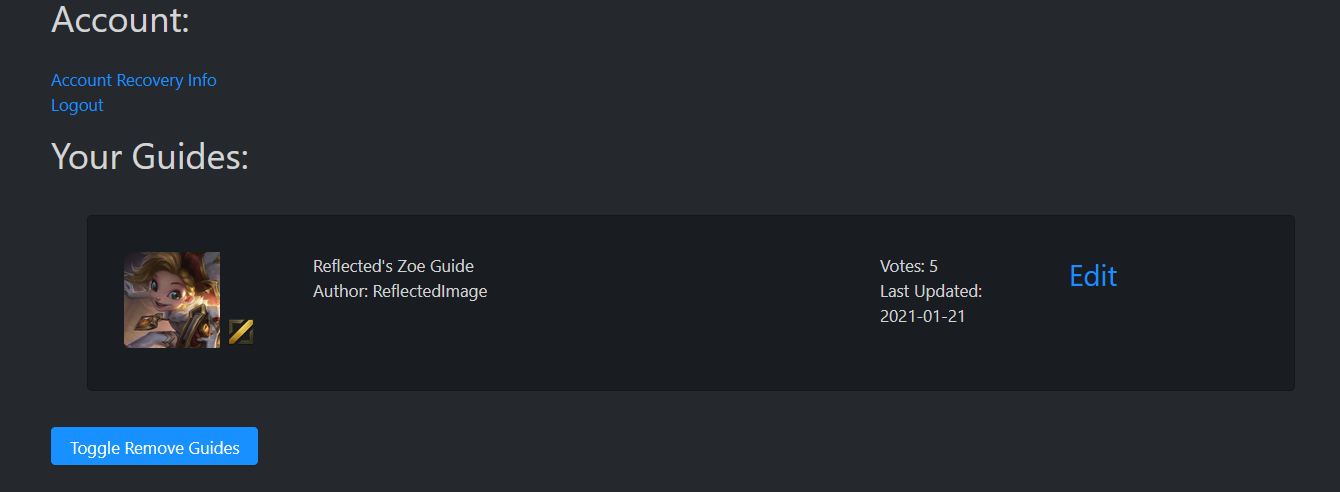
Change log
League of Legends Patch 14.1 - 14.2
Season 14 Added
Added Smolder
League of Legends Patch 13.24 - 13.19
Many many changes
Added Hwei
League of Legends Patch 13.19 - 13.10
Many many changes
Added Briar
League of Legends Patch 13.9 - 13.4
Added Milio
League of Legends Patch 12.22
Site updated with pre-season content
K'Sante added
League of Legends Patch 12.20 - 12.5
Site updated - Nilah Added
League of Legends Patch 12.4
Added Renata Glasc
League of Legends Patch 12.3
Site updated
League of Legends Patch 12.2
League of Legends Patch 12.1
Site updated
League of Legends Patch 11.24
Site updated
League of Legends Patch 11.23
Season 12 Runes & Items added.
League of Legends Patches 11.22 - 11.16
Site updated
LoL Patch 11.15
The site is now fully updated to patch 11.15. Akshan builds have been added. ADC builds now use higher enemy armor and magic resist values to simulate fighting tanks, which makes lethality based builds less likely.
LoL Patch 11.14
The site is now fully updated to patch 11.14.
Aram now available
Builds can be converted to Aram by selecting under Force Starter Items.
Increasing slider ranges
The max range of sliders can now be increased by clicking Double Max Slider Lengths under Advanced Engine Options. This is useful for creating builds such as Maximum Ability Haste builds.
LoL Patch 11.13
Anathema's Chains and Hullbreaker added to the site.
Range sliders for stats
Switching to range sliders for some stats to allow more precision when creating builds. Still a work in progress. Suggestions welcome in Discord.
PBE Dr. Mundo Added
Dr. Mundo has been updated to his reworked version.
Champion Navigation Categories Added
So Lux is an Artillery and Burst champion, Jinx is an Marksman, Viktor is an battlemage, Diana is an Assassin, etc, etc, etc.. You can now directly jump between similar champions at the bottom of the page.
Site Acceleration & Advanced Options
Generating lol builds with custom options is now vastly faster than previously, especially on mobile phones.
Engine options have been moved to the Advanced Options dialog.
Double click Guide Creation
You can now create guides by clicking "Save As Guide" then "Submit Guide" from the build calculator.
User guides have been added to the site
You can now create your own guides on the site. The site's UI has been upgraded using halfmoon. The site is at patch 11.2. Viego will be added tomorrow. You can use the calculator page on the navbar to go directly to the champion build calculators.
LoL build customization added to site
You can now force and block both runes, items. New build settings include changing the mana curve, moving boots, add grievous wounds, restricting gold, unlimited gold, changing combat length, enemy HP/armor/magic resist values and shifting the early game/late game emphasis on stats.
Caveat: The web engine using data files produced by the genetic algorithm to decide which items to check, you need to add additional data files for making extreme changes to builds using the load data file option
Note: it runs faster in Google Chrome
LoL builds updated to 11.1
Site is currently at patch 11.1 (latest).
LoL builds updated to 10.25
Site is currently at patch 10.25 (latest).
PBE Rell on site
Added PBE Rell to the site. Site is currently at patch 10.24 (latest).
Season 11 LoL builds
We have just finished creating the Season 11 lol builds. Season 11 builds are now available here
Lolsolved.gg Initial Release
The League of Legends builds on this site are generated via generic algorithms searching for the theoretical maximum builds for the champions in the various roles. Aka. If Cloak of Agility is buffed then we can tell you how many you should be building and when before anyone has ever played a game with the change.
There is information on the About Page about how this site works or you can start
playing with the Builds here.
We will be starting on season 11 builds shortly.